Au cours des dernières décennies, les chercheurs ont mis au point des méthodes informatiques novatrices pour aider les viticulteurs à résoudre leurs problèmes, principalement liés à la prévision des rendements avant la récolte. L’objectif de cet article est de résumer les travaux de recherche existants liés aux techniques de vision par ordinateur qui traitent de ces questions. Il se concentre sur les approches utilisant des images RGB obtenues directement sur les parcelles, en utilisant différentes méthodes allant des algorithmes classiques de traitement d’image, en passant par les approches d’apprentissage automatique jusqu’à de nouvelles méthodes basées sur l’apprentissage profond. Nous avons l’intention de fournir un examen complet accessible également aux lecteurs non spécialisés, afin de discuter des progrès récents de l’intelligence artificielle dans la viticulture. À cette fin, nous présenterons dans les premières parties les travaux portant sur la détection des fleurs ainsi que des grappes et des raisins, puis dans la dernière partie nous présenterons les différentes méthodes d’estimation du rendement et les problématiques associées.
An early yield prediction is an essential tool for vinegrowers to prepare for the harvest. In the last decades, many methods have been proposed by researchers to solve this problem practically. In particular, recent computer vision advances allow for fruit counting directly in the field. The objective of this publication is to make an exhaustive review of existing works related to grape detection and yield prediction with computer vision algorithms. This review is focused on approaches using RGB proximal images, including classical image processing algorithms, feature extraction, machine learning models, and deep learning. By recalling works on flower counting, grape detection, berry counting, and yield modelization, we highlight challenges on computer vision research for viticulture applications. In addition, we believe that non-specialists can find this review helpful to understand recent advances in artificial intelligence for precision viticulture.
Révisé le :
Accepté le :
Publié le :
Keywords: Viticulture, yield prediction, machine learning, deep learning.
Lucas Mohimont 1 ; Amine Chemchem 2 ; Marine Rondeau 3 ; Mathias Roesler 1 ; François Alin 1 ; Nathalie Gaveau 3 ; Luiz Angelo Steffenel 1
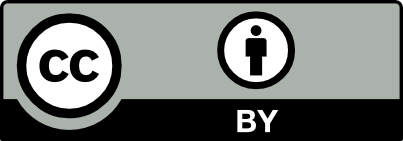
@article{ROIA_2021__2_1_33_0, author = {Lucas Mohimont and Amine Chemchem and Marine Rondeau and Mathias Roesler and Fran\c{c}ois Alin and Nathalie Gaveau and Luiz Angelo Steffenel}, title = {Traitement {d{\textquoteright}Images} et {Apprentissage} {Automatique} pour la {Viticulture} de {Pr\'ecision}}, journal = {Revue Ouverte d'Intelligence Artificielle}, pages = {33--63}, publisher = {Association pour la diffusion de la recherche francophone en intelligence artificielle}, volume = {2}, number = {1}, year = {2021}, doi = {10.5802/roia.9}, language = {fr}, url = {https://roia.centre-mersenne.org/articles/10.5802/roia.9/} }
TY - JOUR AU - Lucas Mohimont AU - Amine Chemchem AU - Marine Rondeau AU - Mathias Roesler AU - François Alin AU - Nathalie Gaveau AU - Luiz Angelo Steffenel TI - Traitement d’Images et Apprentissage Automatique pour la Viticulture de Précision JO - Revue Ouverte d'Intelligence Artificielle PY - 2021 SP - 33 EP - 63 VL - 2 IS - 1 PB - Association pour la diffusion de la recherche francophone en intelligence artificielle UR - https://roia.centre-mersenne.org/articles/10.5802/roia.9/ DO - 10.5802/roia.9 LA - fr ID - ROIA_2021__2_1_33_0 ER -
%0 Journal Article %A Lucas Mohimont %A Amine Chemchem %A Marine Rondeau %A Mathias Roesler %A François Alin %A Nathalie Gaveau %A Luiz Angelo Steffenel %T Traitement d’Images et Apprentissage Automatique pour la Viticulture de Précision %J Revue Ouverte d'Intelligence Artificielle %D 2021 %P 33-63 %V 2 %N 1 %I Association pour la diffusion de la recherche francophone en intelligence artificielle %U https://roia.centre-mersenne.org/articles/10.5802/roia.9/ %R 10.5802/roia.9 %G fr %F ROIA_2021__2_1_33_0
Lucas Mohimont; Amine Chemchem; Marine Rondeau; Mathias Roesler; François Alin; Nathalie Gaveau; Luiz Angelo Steffenel. Traitement d’Images et Apprentissage Automatique pour la Viticulture de Précision. Revue Ouverte d'Intelligence Artificielle, Volume 2 (2021) no. 1, pp. 33-63. doi : 10.5802/roia.9. https://roia.centre-mersenne.org/articles/10.5802/roia.9/
[1] Potential of on-board colour imaging for in-field detection and counting of grape bunches at early fruiting stages, Advances in Animal Biosciences, Volume 8 (2017) no. 22, pp. 505-509 | DOI
[2] A Bayesian framework for joint structure and colour based pixel-wise classification of grapevine proximal images, Computers and Electronics in Agriculture, Volume 158 (2019), pp. 345-357 | DOI
[3] vitisBerry : An Android-smartphone application to early evaluate the number of grapevine berries by means of image analysis, Computers and Electronics in Agriculture, Volume 148 (2018), pp. 19-28 | DOI
[4] A new methodology for estimating the grapevine-berry number per cluster using image analysis, Biosystems Engineering, Volume 156 (2017), pp. 80-95 | DOI
[5] Automated early yield prediction in vineyards from on-the-go image acquisition, Computers and Electronics in Agriculture, Volume 144 (2018), pp. 26-36 | DOI
[6] vitisFlower : Development and Testing of a Novel Android-Smartphone Application for Assessing the Number of Grapevine Flowers per Inflorescence Using Artificial Vision Techniques, Sensors, Volume 15 (2015) no. 9, pp. 21204-21218 | DOI
[7] Grapevine flower estimation by applying artificial vision techniques on images with uncontrolled scene and multi-model analysis, Computers and Electronics in Agriculture, Volume 119 (2015), pp. 92-104 | DOI
[8] Prediction of grape yields from time-series vegetation indices using satellite remote sensing and a machine-learning approach, Remote Sensing Applications : Society and Environment, Volume 22 (2021), 100485 https://www.sciencedirect.com/science/article/pii/S2352938521000215 | DOI
[9] Review. Precision Viticulture. Research topics, challenges and opportunities in site-specific vineyard management, Spanish Journal of Agricultural Research, Volume 7 (2009), pp. 779-790 | DOI
[10] La variabilité des milieux, une réalité : de la région à la plante, Perspectives Agricoles (1997) no. 222, pp. 8-12 | DOI | HAL
[11] Estimation of mango crop yield using image analysis – Segmentation method, Computers and Electronics in Agriculture, Volume 91 (2013), pp. 57-64 https://www.sciencedirect.com/science/article/pii/S0168169912002669 | DOI
[12] Image Segmentation for Fruit Detection and Yield Estimation in Apple Orchards, Journal of Field Robotics, Volume 34 (2016), pp. 1039-1060 | DOI
[13] A Practical Method for Counting Berries based on Image Analysis, Proceedings of the 2nd Annual National Viticulture Research Conference (2008), pp. 4-5 | DOI
[14] A robust algorithm based on color features for grape cluster segmentation, Computers and Electronics in Agriculture, Volume 142 (2017), pp. 41-49 | DOI
[15] Weakly Supervised Fruit Counting for Yield Estimation Using Spatial Consistency, IEEE Robotics and Automation Letters, Volume 4 (2019) no. 3, pp. 2348-2355 | DOI
[16] Grape clusters and foliage detection algorithms for autonomous selective vineyard sprayer, Intelligent Service Robotics, Volume 3 (2010) no. 44, pp. 233-243 | DOI
[17] A Robot System for Pruning Grape Vines, Journal of Field Robotics, Volume 34 (2017) no. 6, pp. 1100-1122 https://onlinelibrary.wiley.com/doi/abs/10.1002/rob.21680 | DOI
[18] Emerging Properties in Self-Supervised Vision Transformers (2021) (https://arxiv.org/abs/2104.14294)
[19] Using Feedforward Neural Networks for Color Based Grape Detection in Field Images, CSCUBS 2016 - Computer Science Conference for University of Bonn Students (2016), pp. 23-33 /paper/Using-Feedforward-Neural-Networks-for-Color-Based-Casser/139ccba0b3a00565f61febcc62f98c6c44cca990 | DOI
[20] Grape detection with convolutional neural networks, Expert Systems with Applications, Volume 159 (2020), 113588 | DOI
[21] Grape Detection By Image Processing, IECON 2006 - 32nd Annual Conference on IEEE Industrial Electronics (2006), pp. 3697-3702 | DOI
[22] Counting Apples and Oranges With Deep Learning : A Data-Driven Approach, IEEE Robotics and Automation Letters, Volume 2 (2017) no. 2, pp. 781-788 | DOI
[23] Early Yield Prediction Using Image Analysis of Apple Fruit and Tree Canopy Features with Neural Networks, Journal of Imaging, Volume 3 (2017) no. 1, 6, 13 pages https://www.mdpi.com/2313-433X/3/1/6 | DOI
[24] Crop development, crop estimation and crop control to secure quality and production of major wine grape varieties : a national approach, Adelaide, Grape and Wine Research and Development Corporation, 2001 | DOI
[25] Characterization of Vineyard’s Canopy through Fuzzy Clustering and SVM over Color Images, 3rd CIGR International Conference of Agricultural Engineering (CIGR-AgEng2012), Volume 1 (2012), 6 pages | DOI
[26] GBCNet : In-Field Grape Berries Counting for Yield Estimation by Dilated CNNs, Applied Sciences, Volume 10 (2020) no. 1414, 4870, 15 pages | DOI
[27] Classification of plant structures from uncalibrated image sequences, 2012 IEEE Workshop on the Applications of Computer Vision (WACV) (2012), pp. 329-336 | DOI
[28] A Low-Cost and Unsupervised Image Recognition Methodology for Yield Estimation in a Vineyard, Frontiers in Plant Science, Volume 10 (2019), 559 https://www.frontiersin.org/article/10.3389/fpls.2019.00559 | DOI
[29] On-the-go assessment of vineyard canopy porosity, bunch and leaf exposure by image analysis, Australian Journal of Grape and Wine Research, Volume 25 (2019) no. 3, pp. 363-374 | DOI
[30] Assessment of flower number per inflorescence in grapevine by image analysis under field conditions, Journal of the Science of Food and Agriculture, Volume 94 (2014) no. 10, pp. 1981-1987 | DOI
[31] Assessment of cluster yield components by image analysis, Journal of the Science of Food and Agriculture, Volume 95 (2015) no. 66, pp. 1274-1282 | DOI
[32] Grapevine Yield and Leaf Area Estimation Using Supervised Classification Methodology on RGB Images Taken under Field Conditions, Sensors, Volume 12 (2012) no. 12, pp. 16988-17006 | DOI
[33] Detection of grapes in natural environment using feedforward neural network as a classifier, 2016 SAI Computing Conference (SAI) (2016), pp. 1330-1334 | DOI
[34] An yield estimation in citrus orchards via fruit detection and counting using image processing, Computers and Electronics in Agriculture, Volume 140 (2017), pp. 103-112 https://www.sciencedirect.com/science/article/pii/S0168169916312455 | DOI
[35] Yield prediction from digital image analysis : A technique with potential for vineyard assessments prior to harvest, Australian Journal of Grape and Wine Research, Volume 10 (2004) no. 33, pp. 196-198 | DOI
[36] Vineyard Yield Estimation Based on the Analysis of High Resolution Images Obtained with Artificial Illumination at Night, Sensors, Volume 15 (2015) no. 4, pp. 8284-8301 | DOI
[37] State-of-the-Art Convolutional Neural Networks for Smart Farms : A Review, Intelligent Computing (2019), pp. 763-775 | DOI
[38] An adaptable approach to automated visual detection of plant organs with applications in grapevine breeding, Biosystems Engineering, Volume 183 (2019), pp. 170-183 | DOI
[39] A Camera and Laser System for Automatic Vine Balance Assessment, American Society of Agricultural and Biological Engineers Annual International Meeting 2011, ASABE 2011, Volume 7 (2011) | DOI
[40] Early Estimation of Vineyard Yield : site specific counting of berries by using a smartphone, International Conference on Agiculture Engineering (AgEng) (2012), 143 | DOI | HAL
[41] Investigating 2-D and 3-D Proximal Remote Sensing Techniques for Vineyard Yield Estimation, Sensors, Volume 19 (2019) no. 17, 3652, 20 pages | DOI
[42] Mask R-CNN (2018) (https://arxiv.org/abs/1703.06870)
[43] Yield Prognosis for the Agrarian Management of Vineyards using Deep Learning for Object Counting, Wirtschaftsinformatik 2019 Proceedings (2019), pp. 407-421 https://aisel.aisnet.org/wi2019/track05/papers/3 | DOI
[44] Vineyard yield estimation by automatic 3D bunch modelling in field conditions, Computers and Electronics in Agriculture, Volume 110 (2015), pp. 17-26 | DOI
[45] Assessment of grape cluster yield components based on 3D descriptors using stereo vision, Food Control, Volume 50 (2015), pp. 273-282 | DOI
[46] Deep learning in agriculture : A survey, Computers and Electronics in Agriculture, Volume 147 (2018), pp. 70-90 https://www.sciencedirect.com/science/article/pii/S0168169917308803 | DOI
[47] Real-time Fruit Detection Using Deep Neural Networks, 14th International Conference on Precision Agriculture (2018) | DOI | HAL
[48] Vineyard Vigilant and INNovative Ecological Rover (VVINNER) : an autonomous robot for automated scoring of vineyards, International Conference of Agricultural Engineering (2014), 2098 | DOI | HAL
[49] Vine disease detection in UAV multispectral images using optimized image registration and deep learning segmentation approach, Computers and Electronics in Agriculture, Volume 174 (2020), p. 105446 https://www.sciencedirect.com/science/article/pii/S016816991932558X | DOI
[50] Phenoliner : A New Field Phenotyping Platform for Grapevine Research, Sensors, Volume 17 (2017) no. 77, 1625, 18 pages | DOI
[51] Phenoliner : A New Field Phenotyping Platform for Grapevine Research, Sensors, Volume 17 (2017) no. 7, 1625, 18 pages https://www.mdpi.com/1424-8220/17/7/1625 | DOI
[52] An Automated Field Phenotyping Pipeline for Application in Grapevine Research, Sensors, Volume 15 (2015) no. 3, pp. 4823-4836 https://www.mdpi.com/1424-8220/15/3/4823 | DOI
[53] BAT (Berry Analysis Tool) : A high-throughput image interpretation tool to acquire the number, diameter, and volume of grapevine berries, Vitis -Geilweilerhof-, Volume 52 (2013), pp. 129-135 | DOI
[54] Field phenotyping of grapevine growth using dense stereo reconstruction, BMC Bioinformatics, Volume 16 (2015) no. 1, 143 | DOI
[55] ImageNet Classification with Deep Convolutional Neural Networks, Proceedings of the 25th International Conference on Neural Information Processing Systems - Volume 1 (NIPS’12) (2012), pp. 1097-1105 | DOI
[56] In-Field Grape Cluster Size Assessment for Vine Yield Estimation Using a Mobile Robot and a Consumer Level RGB-D Camera, IEEE Robotics and Automation Letters, Volume 5 (2020) no. 2, 6, pp. 2031-2038 | DOI
[57] Handwritten Digit Recognition with a Back-Propagation Network (1990), pp. 396-404 http://papers.nips.cc/paper/293-handwritten-digit-recognition-with-a-back-propagation-network.pdf | DOI
[58] 3DBunch : A Novel iOS-Smartphone Application to Evaluate the Number of Grape Berries per Bunch Using Image Analysis Techniques, IEEE Access, Volume 8 (2020), pp. 114663-114674 | DOI
[59] A computer vision system for early stage grape yield estimation based on shoot detection, Computers and Electronics in Agriculture, Volume 137 (2017), 1625, pp. 88-101 | DOI
[60] A robust automated flower estimation system for grape vines, Biosystems Engineering, Volume 172 (2018), 1625, pp. 110-123 https://www.sciencedirect.com/science/article/pii/S1537511017304610 | DOI
[61] Towards Automated Yield Estimation in Viticulture, Proceedings of the Australasian Conference on Robotics and Automation (2013), 9 pages | DOI
[62] Automatic grape bunch detection in vineyards with an SVM classifier, Journal of Applied Logic, Volume 13 (2015) no. 4, Part 34, Part 3, pp. 643-653 | DOI
[63] A Lightweight Method for Grape Berry Counting based on Automated 3 D Bunch Reconstruction from a Single Image, ICRA, International Conference on Robotics and Automation (IEEE), Workshop on Robotics in Agriculture (2015) | DOI
[64] A vision-based robust grape berry counting algorithm for fast calibration-free bunch weight estimation in the field, Computers and Electronics in Agriculture, Volume 173 (2020), 105360, 11 pages | DOI
[65] Monocular Camera Based Fruit Counting and Mapping With Semantic Data Association, IEEE Robotics and Automation Letters, Volume 4 (2019) no. 3, 465, pp. 2296-2303 | DOI
[66] A Wireless Sensor Network for Vineyard Monitoring That Uses Image Processing, Sensors, Volume 11 (2011) no. 6, 905, pp. 6165-6196 https://www.mdpi.com/1424-8220/11/6/6165 | DOI
[67] Using an Unmanned Ground Vehicle to Scout Vineyards for Non-intrusive Estimation of Canopy Features and Grape Yield, 20th GiESCO International Meeting (2017)
[68] Design of a Vineyard Terrestrial Robot for Multiple Applications as Part of the Innovation of Process and Product : Preliminary Results, 2020 IEEE International Conference on Engineering Veracruz (ICEV) (2020), pp. 1-4 | DOI
[69] A vision methodology for harvesting robot to detect cutting points on peduncles of double overlapping grape clusters in a vineyard, Computers in Industry, Volume 99 (2018), pp. 130-139 https://www.sciencedirect.com/science/article/pii/S0166361517305298 | DOI
[70] Robust Grape Cluster Detection in a Vineyard by Combining the AdaBoost Framework and Multiple Color Components, Sensors, Volume 16 (2016) no. 12, 2098, 20 pages https://www.ncbi.nlm.nih.gov/pmc/articles/PMC5191078/ | DOI
[71] Vision-based extraction of spatial information in grape clusters for harvesting robots, Biosystems Engineering, Volume 151 (2016), pp. 90-104 https://www.sciencedirect.com/science/article/pii/S1537511015303901 | DOI
[72] High-precision 3D detection and reconstruction of grapes from laser range data for efficient phenotyping based on supervised learning, Computers and Electronics in Agriculture, Volume 135 (2017), pp. 300-311 https://www.sciencedirect.com/science/article/pii/S0168169916308602 | DOI
[73] Automatic green fruit counting in orange trees using digital images, Computers and Electronics in Agriculture, Volume 127 (2016), pp. 572-581 https://www.sciencedirect.com/science/article/pii/S0168169916305294 | DOI
[74] Deep neural networks for grape bunch segmentation in natural images from a consumer-grade camera, Precision Agriculture (2021), pp. 387-413 | DOI
[75] Practical Applications of a Multisensor UAV Platform Based on Multispectral, Thermal and RGB High Resolution Images in Precision Viticulture, Agriculture, Volume 8 (2018) no. 7, 116, 13 pages https://www.mdpi.com/2077-0472/8/7/116 | DOI
[76] Technology in precision viticulture : a state of the art review, International Journal of Wine Research, Volume 7 (2015), pp. 69-81 https://www.dovepress.com/technology-in-precision-viticulture-a-state-of-the-art-review-peer-reviewed-article-IJWR | DOI
[77] In-field high throughput grapevine phenotyping with a consumer-grade depth camera, Computers and Electronics in Agriculture, Volume 156 (2019), pp. 293-306 | DOI
[78] Image analysis-based modelling for flower number estimation in grapevine, Journal of the Science of Food and Agriculture, Volume 97 (2017) no. 3, 116, pp. 784-792 | DOI
[79] On-the-Go Grapevine Yield Estimation Using Image Analysis and Boolean Model, Journal of Sensors, Volume 2018 (2018), 9634752, 15 pages | DOI
[80] Implementation of Hough transform for fruit image segmentation, Procedia Engineering, Volume 35 (2012), pp. 230-239 | DOI
[81] ROLS : Robust Object-Level SLAM for Grape Counting, 2019 IEEE/CVF Conference on Computer Vision and Pattern Recognition Workshops (CVPRW) (2019), pp. 2648-2656 | DOI
[82] Yield estimation in vineyards by visual grape detection, 2011 IEEE/RSJ International Conference on Intelligent Robots and Systems (2011), 969, pp. 2352-2358 | DOI
[83] Automated Visual Yield Estimation in Vineyards, J. Field Robot., Volume 31 (2014) no. 55, pp. 837-860 | DOI
[84] A Non-Invasive Method Based on Computer Vision for Grapevine Cluster Compactness Assessment Using a Mobile Sensing Platform under Field Conditions, Sensors, Volume 19 (2019) no. 17, 3799 https://www.ncbi.nlm.nih.gov/pmc/articles/PMC6749308/ | DOI
[85] VineSens : An Eco-Smart Decision-Support Viticulture System, Sensors, Volume 17 (2017) no. 3, 465, 26 pages | DOI
[86] A pattern recognition strategy for visual grape bunch detection in vineyards, Computers and Electronics in Agriculture, Volume 151 (2018), pp. 136-149 | DOI
[87] eAGROBOT - A robot for early crop disease detection using image processing, 2014 International Conference on Electronics and Communication Systems (ICECS) (2014), 559, pp. 1-6 | DOI
[88] Grape berry calibration by computer vision using elliptical model fitting, ECPA 2007, 6th European Conference on Precision Agriculture (2007), pp. 581-587 | DOI | HAL
[89] Identification of mature grape bunches using image processing and computational intelligence methods, 2014 IEEE Symposium on Computational Intelligence for Multimedia, Signal and Vision Processing (CIMSIVP) (2014), pp. 1-6 | DOI
[90] Deep Count : Fruit Counting Based on Deep Simulated Learning, Sensors, Volume 17 (2017) no. 4, 905, 12 pages https://www.mdpi.com/1424-8220/17/4/905 | DOI
[91] Automatic detection of bunches of grapes in natural environment from color images, Journal of Applied Logic, Volume 10 (2012) no. 44, pp. 285-290 | DOI
[92] U-Net : Convolutional Networks for Biomedical Image Segmentation, Medical Image Computing and Computer-Assisted Intervention – MICCAI 2015 (Lecture Notes in Computer Science) (2015), pp. 234-241 | DOI
[93] Automated image analysis framework for high-throughput determination of grapevine berry sizes using conditional random fields, Computers and Electronics in Agriculture, Volume 100 (2014), pp. 148-158 | DOI
[94] Towards Automated Large-Scale 3D Phenotyping of Vineyards under Field Conditions, Sensors, Volume 16 (2016) no. 12, 2136, 25 pages | DOI
[95] Efficient identification, localization and quantification of grapevine inflorescences in unprepared field images using Fully Convolutional Networks, Journal of Grapevine Research, Volume 58 (2019) no. 3, 3799, pp. 95-104 | DOI
[96] Automatic grape bunch detection in vineyards based on affordable 3D phenotyping using a consumer webcam, XI Congresso Brasileiro de Agroinformática (SBIAgro 2017), 2017 | DOI
[97] Grape detection, segmentation, and tracking using deep neural networks and three-dimensional association, Computers and Electronics in Agriculture, Volume 170 (2020), 105247 | DOI
[98] Computer Vision and Machine Learning for Viticulture Technology, IEEE Access, Volume 6 (2018), pp. 67494-67510 | DOI
[99] Fully Convolutional Networks for Semantic Segmentation, IEEE Transactions on Pattern Analysis and Machine Intelligence, Volume 39 (2017) no. 4, pp. 640-651 | DOI
[100] In Vino Veritas : Estimating Vineyard Grape Yield from Images Using Deep Learning, Advances in Artificial Intelligence (Lecture Notes in Computer Science) (2019), 4870, pp. 212-224 | DOI
[101] DeepGrapes : Precise Detection of Grapes in Low-resolution Images, IFAC-PapersOnLine, Volume 51 (2018) no. 66, 113588, pp. 185-189 | DOI
[102] Robust Grape Detector Based on SVMs and HOG Features, Computational Intelligence and Neuroscience, Volume 2017 (2017), 3478602 | DOI
[103] Automatic fruit recognition and counting from multiple images, Biosystems Engineering, Volume 118 (2014), pp. 203-215 https://www.sciencedirect.com/science/article/pii/S1537511013002109 | DOI
[104] Automatic Flower Number Evaluation in Grapevine Inflorescences Using RGB Images, American Journal of Enology and Viticulture, Volume 71 (2019), pp. 10-16 https://www.ajevonline.org/content/early/2019/09/12/ajev.2019.19036 | DOI
[105] Comparison of convolutional neural networks in fruit detection and counting : A comprehensive evaluation, Computers and Electronics in Agriculture, Volume 173 (2020), 105348 https://www.sciencedirect.com/science/article/pii/S016816991932232X | DOI
[106] Attention is All you Need, Advances in Neural Information Processing Systems, Volume 30 (2017), 105360 https://proceedings.neurips.cc/paper/2017/file/3f5ee243547dee91fbd053c1c4a845aa-Paper.pdf | DOI
[107] Grapevine yield prediction using image analysis – improving the estimation of non-visible bunches, European Federation for Information Technology in Agriculture, Food and the Environment (EFITA) (2019), 105247, p. 6 | DOI
[108] Automated Crop Yield Estimation for Apple Orchards (2013), 105348, pp. 745-758 | DOI
[109] Green Grape Detection and Picking-Point Calculation in a Night-Time Natural Environment Using a Charge-Coupled Device (CCD) Vision Sensor with Artificial Illumination, Sensors, Volume 18 (2018) no. 4, 969, 17 pages https://www.ncbi.nlm.nih.gov/pmc/articles/PMC5948586/ | DOI
[110] Counting of grapevine berries in images via semantic segmentation using convolutional neural networks, ISPRS Journal of Photogrammetry and Remote Sensing, Volume 164 (2020), 100485, pp. 73-83 | DOI
[111] Design and Experiment of Intelligent Grape Bagging Robot, Applied Mechanics and Materials, Volume 389 (2013), pp. 706-711 | DOI
[112] L’agriculture de précision, Ingénieries eau-agriculture-territoires (1997) no. 12, pp. 67-79 | DOI | HAL
Cité par Sources :