Dans la thématique grandissante de la reconnaissance d’activités de la vie quotidienne au sein de maisons intelligentes, les réseaux de neurones basées sur les Long Short Term Memory (LSTM) ont démontré leur efficacité. En étudiant l’ordre des activations des capteurs et leurs dépendances temporelles, on traduit les actions humaines comme une suite d’événements dans le temps plus ou moins corrélés. Cependant, l’activité humaine n’est pas une suite d’actions dénuées de sens ni de contexte. Nous proposons d’utiliser et de comparer deux méthodes provenant du traitement du langage naturel pour, justement, prendre en compte la sémantique et le contexte des capteurs afin d’améliorer les algorithmes dans les tâches de classification de séquences d’activités : Word2Vec, un embedding de sémantique statique, et ELMo, un embedding contextuel. Les résultats, sur des datasets réels de maisons intelligentes, indiquent que cette approche fournit des informations utiles, comme une carte de l’organisation des capteurs, et réduit par ailleurs la confusion entre les classes d’activités quotidiennes. Elle permet d’obtenir de meilleures performances sur des datasets contenant des activités concurrentes avec plusieurs résidents ou des animaux domestiques. Nos tests montrent également que les embeddings peuvent être pré-entraînés sur des datasets différents du jeu de données cible, permettant ainsi un apprentissage par transfert. Nous démontrons ainsi que la prise en compte du contexte et de la sémantique des capteurs augmente les performances de classification des algorithmes et permet l’apprentissage par transfert.
Neural networks based on Long Short Term Memory (LSTM) have demonstrated their efficiency in the growing field of recognition of daily life activities in smart homes,. By studying the sensor activations order and their temporal dependencies, human actions are translated as a sequence of more or less correlated events in time. However, human activity is not a sequence of actions without meaning and context. We propose to use and compare two methods coming from natural language processing to take into account the semantics and context of sensors in order to improve algorithms in activity sequence classification: Word2Vec, a static semantic embedding, and ELMo, a contextual embedding. The results, on real smart home datasets, indicate that this approach provides useful information, such as a map of sensor organization, and also reduces confusion between classes of daily activities. It achieves better performance on datasets containing concurrent activities with multiple residents or pets. Our tests also show that embeddings can be pre-trained on datasets that are different from the target dataset, thus allowing transfer learning. We thus demonstrate that taking into account the context and semantics of the sensors increases the classification performance of the algorithms and enables transfer learning.
Accepté le :
Publié le :
Damien Bouchabou 1 ; Sao Mai Nguyen 1 ; Christophe Lohr 1 ; Ioannis Kanellos 1 ; Benoit LeDuc 2
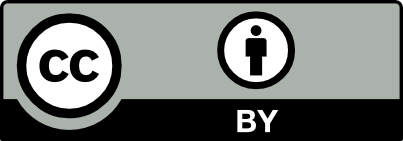
@article{ROIA_2023__4_1_129_0, author = {Damien Bouchabou and Sao Mai Nguyen and Christophe Lohr and Ioannis Kanellos and Benoit LeDuc}, title = {Reconnaissance d{\textquoteright}activit\'es de la vie quotidienne au moyen de capteurs domotiques et d{\textquoteright}apprentissage profond~: lorsque syntaxe, s\'emantique et contexte se rencontrent}, journal = {Revue Ouverte d'Intelligence Artificielle}, pages = {129--156}, publisher = {Association pour la diffusion de la recherche francophone en intelligence artificielle}, volume = {4}, number = {1}, year = {2023}, doi = {10.5802/roia.53}, language = {fr}, url = {https://roia.centre-mersenne.org/articles/10.5802/roia.53/} }
TY - JOUR AU - Damien Bouchabou AU - Sao Mai Nguyen AU - Christophe Lohr AU - Ioannis Kanellos AU - Benoit LeDuc TI - Reconnaissance d’activités de la vie quotidienne au moyen de capteurs domotiques et d’apprentissage profond : lorsque syntaxe, sémantique et contexte se rencontrent JO - Revue Ouverte d'Intelligence Artificielle PY - 2023 SP - 129 EP - 156 VL - 4 IS - 1 PB - Association pour la diffusion de la recherche francophone en intelligence artificielle UR - https://roia.centre-mersenne.org/articles/10.5802/roia.53/ DO - 10.5802/roia.53 LA - fr ID - ROIA_2023__4_1_129_0 ER -
%0 Journal Article %A Damien Bouchabou %A Sao Mai Nguyen %A Christophe Lohr %A Ioannis Kanellos %A Benoit LeDuc %T Reconnaissance d’activités de la vie quotidienne au moyen de capteurs domotiques et d’apprentissage profond : lorsque syntaxe, sémantique et contexte se rencontrent %J Revue Ouverte d'Intelligence Artificielle %D 2023 %P 129-156 %V 4 %N 1 %I Association pour la diffusion de la recherche francophone en intelligence artificielle %U https://roia.centre-mersenne.org/articles/10.5802/roia.53/ %R 10.5802/roia.53 %G fr %F ROIA_2023__4_1_129_0
Damien Bouchabou; Sao Mai Nguyen; Christophe Lohr; Ioannis Kanellos; Benoit LeDuc. Reconnaissance d’activités de la vie quotidienne au moyen de capteurs domotiques et d’apprentissage profond : lorsque syntaxe, sémantique et contexte se rencontrent. Revue Ouverte d'Intelligence Artificielle, Volume 4 (2023) no. 1, pp. 129-156. doi : 10.5802/roia.53. https://roia.centre-mersenne.org/articles/10.5802/roia.53/
[1] Method for Undefined Complex Human Activity Recognition, 2021 International Conference on Industrial Engineering, Applications and Manufacturing (ICIEAM), IEEE (2021), pp. 797-801 | DOI
[2] Localization of humans, objects, and robots interacting on load-sensing floors, IEEE Sensors Journal, Volume 16 (2015) no. 4, pp. 1026-1037 | DOI
[3] A survey on digital twin : definitions, characteristics, applications, and design implications, IEEE access, Volume 7 (2019), pp. 167653-167671 | DOI
[4] Enriching word vectors with subword information, Transactions of the Association for Computational Linguistics, Volume 5 (2017), pp. 135-146 | DOI
[5] Fully Convolutional Network Bootstrapped by Word Encoding and Embedding for Activity Recognition in Smart Homes, IJCAI 2020 Workshop on Deep Learning for Human Activity Recognition, Yokohama, Japan (2021) | DOI
[6] et al. A Survey of Human Activity Recognition in Smart Homes Based on IoT Sensors Algorithms : Taxonomies, Challenges, and Opportunities with Deep Learning, Sensors, Volume 21 (2021) no. 18, 6037 | DOI
[7] et al. Using Language Model to Bootstrap Human Activity Recognition Ambient Sensors Based in Smart Homes, Electronics, Volume 10 (2021) no. 20, 2498 | DOI
[8] Habit2vec : Trajectory semantic embedding for living pattern recognition in population, IEEE Transactions on Mobile Computing, Volume 19 (2019) no. 5, pp. 1096-1108 | DOI
[9] A review of smart homes – Present state and future challenges, Computer methods and programs in biomedicine, Volume 91 (2008) no. 1, pp. 55-81 | DOI
[10] CASAS : A smart home in a box, Computer, Volume 46 (2012) no. 7, pp. 62-69 | DOI
[11] Sensor-based and vision-based human activity recognition : A comprehensive survey, Pattern Recognition, Volume 108 (2020), 107561 | DOI
[12] Sensor-based datasets for human activity recognition – a systematic review of literature, IEEE Access, Volume 6 (2018), pp. 59192-59210 | DOI
[13] World population prospects 2019 : Highlights, United Nations Department for Economic and Social Affairs, New York, NY, 2019
[14] Bert : Pre-training of deep bidirectional transformers for language understanding (2018) (https://arxiv.org/abs/1810.04805)
[15] Deep learning for time series classification : a review, Data Mining and Knowledge Discovery, Volume 33 (2019) no. 4, pp. 917-963 | DOI | MR | Zbl
[16] Unobtrusive activity recognition of elderly people living alone using anonymous binary sensors and DCNN, IEEE journal of biomedical and health informatics, Volume 23 (2019) no. 2, pp. 693-702 | DOI
[17] Efficient activity recognition in smart homes using delayed fuzzy temporal windows on binary sensors, IEEE journal of biomedical and health informatics, Volume 24 (2019) no. 2, pp. 387-395 | DOI
[18] Joint learning of temporal models to handle imbalanced data for human activity recognition, Applied Sciences, Volume 10 (2020) no. 15, 5293 | DOI
[19] Zero-data learning of new tasks, Proceedings of the Twenty-Third AAAI Conference on Artificial Intelligence (AAAI’08), Volume 1 (2008) no. 2, pp. 646-651
[20] A Sequential Deep Learning Application for Recognising Human Activities in Smart Homes, Neurocomputing, Volume 396 (2020), pp. 501-513 | DOI
[21] Network in network (2013) (https://arxiv.org/abs/1312.4400)
[22] Improvements of the xAAL home automation system, Future internet, Volume 12 (2020) no. 6, 104 | DOI
[23] Characterizing word embeddings for zero-shot sensor-based human activity recognition, Sensors, Volume 19 (2019) no. 22, 5043 | DOI
[24] Umap : Uniform manifold approximation and projection for dimension reduction (2018) (https://arxiv.org/abs/1802.03426)
[25] Ensemble classifier of long short-term memory with fuzzy temporal windows on binary sensors for activity recognition, Expert Systems with Applications, Volume 114 (2018), pp. 441-453 | DOI
[26] Distributed representations of words and phrases and their compositionality (2013) (https://arxiv.org/abs/1310.4546)
[27] Employing a deep convolutional neural network for human activity recognition based on binary ambient sensor data, Proceedings of the 13th ACM International Conference on PErvasive Technologies Related to Assistive Environments (2020), pp. 1-7 | DOI
[28] Complete Cross-Validation for Nearest Neighbor Classifiers, Proceedings of the Seventeenth International Conference on Machine Learning (ICML ’00), Morgan Kaufmann Publishers Inc., San Francisco, CA, USA (2000), p. 639–646
[29] Glove : Global vectors for word representation, Proceedings of the 2014 conference on empirical methods in natural language processing (EMNLP) (2014), pp. 1532-1543 | DOI
[30] Deep contextualized word representations (2018) (https://arxiv.org/abs/1802.05365)
[31] Virtualhome : Simulating household activities via programs, Proceedings of the IEEE Conference on Computer Vision and Pattern Recognition (2018), pp. 8494-8502 | DOI
[32] Improving language understanding by generative pre-training, 2018
[33] Recognition of activities of daily living using home automation sensors and deep learning context and semantic, https://github.com/dbouchabou/HAR-Context-and-Semantic.git (Accessed : 2022-03-14)
[34] Software Framework for Topic Modelling with Large Corpora, Proceedings of the LREC 2010 Workshop on New Challenges for NLP Frameworks, ELRA, Valletta, Malta (2010), pp. 45-50 (http://is.muni.cz/publication/884893/en) | DOI
[35] Description and validation of a circular padding method for linear roughness measurements of short data lengths, MethodsX, Volume 7 (2020), 101122 | DOI
[36] Evaluating machine learning techniques for activity classification in smart home environments, International Journal of Information Systems and Computer Sciences, Volume 12 (2018) no. 2, pp. 48-54
[37] Neural machine translation of rare words with subword units (2015) (https://arxiv.org/abs/1508.07909)
[38] Combining Public Machine Learning Models by Using Word Embedding for Human Activity Recognition, 2021 IEEE International Conference on Pervasive Computing and Communications Workshops and other Affiliated Events (PerCom Workshops), IEEE (2021), pp. 2-7 | DOI
[39] Convolutional and recurrent neural networks for activity recognition in smart environment, Towards integrative machine learning and knowledge extraction, Springer, 2017, pp. 194-205 | DOI
[40] Human activity recognition using recurrent neural networks, International Cross-Domain Conference for Machine Learning and Knowledge Extraction, Springer (2017), pp. 267-274 | DOI
[41] Smart cities and the ageing population, The 32nd Meeting of WWRF (2014)
[42] Multi-resident activity recognition in a smart home using RGB activity image and DCNN, IEEE Sensors Journal, Volume 18 (2018) no. 23, pp. 9718-9727 | DOI
[43] et al. Neurological rehabilitation, Elsevier Health Sciences, 2012
[44] Activities of Daily Living Recognition With Binary Environment Sensors Using Deep Learning : A Comparative Study, IEEE Sensors Journal, Volume 21 (2020) no. 4, pp. 5423-5433 | DOI
[45] Time series classification from scratch with deep neural networks : A strong baseline, 2017 International joint conference on neural networks (IJCNN), IEEE (2017), pp. 1578-1585 | DOI
[46] Convolution with even-sized kernels and symmetric padding (2019) (https://arxiv.org/abs/1903.08385)
[47] et al. Google’s neural machine translation system : Bridging the gap between human and machine translation (2016) (https://arxiv.org/abs/1609.08144)
Cité par Sources :