La ville intelligente s’intéresse à l’amélioration de la qualité de vie de ses habitants. De nombreux capteurs ad hoc nécessitent alors d’être déployés pour connaître l’état de l’environnement dans lequel les activités humaines se déroulent. Si ces capteurs sont souvent bon marché, leurs coûts d’installation et de maintenance augmentent rapidement avec leur nombre. La problématique adressée dans ce papier consiste à estimer des informations environnementales dans le cas où des capteurs physiques ne sont pas disponibles, pour limiter les coûts engendrés par l’installation et la maintenance de capteurs supplémentaires.
Le système HybridIoT permet d’estimer les valeurs environnementales manquantes dans des réseaux de capteurs à grande échelle à l’aide de mécanismes distincts : (i) une estimation endogène à partir d’un historique de données, (ii) une estimation endogène à l’aide de capteurs voisins homogènes et (iii) une estimation exogène. Ce papier s’intéresse à une amélioration de la technique d’estimation à l’aide de capteurs voisins homogènes (ii). Plus concrètement, notre contribution est triple : la définition d’une nouvelle approche géospatiale pour estimer des valeurs manquantes dans des environnements à grande échelle, l’évaluation de cette méthode géospatiale pour estimer des valeurs environnementales sur la ville de Toulouse, et enfin les premières avancées sur le déploiement du système dans le cadre du Groupement d’Interêt Scientifique (GIS) neOCampus.
The smart city aims at improving the quality of life of its citizens. Many sensors have to be deployed to monitor the state of the environment in which human activities take place. Despite these sensors being often cheap, their installation and maintenance costs increase rapidly with their number. In this paper, we address the problem of estimating environmental information where physical sensors are not available, to limit the costs related to the installation of additional sensors.
This paper presents the HybridIoT system for estimating missing environmental values in large-scale sensor networks. Our contribution is threefold: the definition of an approach for estimating missing values in large-scale environments, the definition and evaluation of a new geospatial method for estimating environmental values in the city of Toulouse, and finally the initial progress on deploying the system as part of the GIS neOCampus.
Révisé le :
Accepté le :
Publié le :
Mots clés : Smart City, Cooperative Multi-Agent Systems, Missing Data Estimation.
Davide Andrea Guastella 1 ; Valérie Camps 2 ; Marie-Pierre Gleizes 2
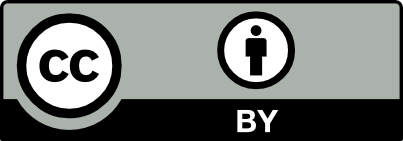
@article{ROIA_2024__5_1_63_0, author = {Davide Andrea Guastella and Val\'erie Camps and Marie-Pierre Gleizes}, title = {Estimation d{\textquoteright}informations environnementales avec le syst\`eme {HybridIoT~~:} un cas d{\textquoteright}\'etude sur la ville de {Toulouse}}, journal = {Revue Ouverte d'Intelligence Artificielle}, pages = {63--91}, publisher = {Association pour la diffusion de la recherche francophone en intelligence artificielle}, volume = {5}, number = {1}, year = {2024}, doi = {10.5802/roia.65}, language = {fr}, url = {https://roia.centre-mersenne.org/articles/10.5802/roia.65/} }
TY - JOUR AU - Davide Andrea Guastella AU - Valérie Camps AU - Marie-Pierre Gleizes TI - Estimation d’informations environnementales avec le système HybridIoT : un cas d’étude sur la ville de Toulouse JO - Revue Ouverte d'Intelligence Artificielle PY - 2024 SP - 63 EP - 91 VL - 5 IS - 1 PB - Association pour la diffusion de la recherche francophone en intelligence artificielle UR - https://roia.centre-mersenne.org/articles/10.5802/roia.65/ DO - 10.5802/roia.65 LA - fr ID - ROIA_2024__5_1_63_0 ER -
%0 Journal Article %A Davide Andrea Guastella %A Valérie Camps %A Marie-Pierre Gleizes %T Estimation d’informations environnementales avec le système HybridIoT : un cas d’étude sur la ville de Toulouse %J Revue Ouverte d'Intelligence Artificielle %D 2024 %P 63-91 %V 5 %N 1 %I Association pour la diffusion de la recherche francophone en intelligence artificielle %U https://roia.centre-mersenne.org/articles/10.5802/roia.65/ %R 10.5802/roia.65 %G fr %F ROIA_2024__5_1_63_0
Davide Andrea Guastella; Valérie Camps; Marie-Pierre Gleizes. Estimation d’informations environnementales avec le système HybridIoT : un cas d’étude sur la ville de Toulouse. Revue Ouverte d'Intelligence Artificielle, Volume 5 (2024) no. 1, pp. 63-91. doi : 10.5802/roia.65. https://roia.centre-mersenne.org/articles/10.5802/roia.65/
[1] Indoor Air-Temperature Forecast for Energy-Efficient Management in Smart Buildings, 2018 IEEE Int. Conference on Environment and Electrical Engineering (2018), pp. 1-6 | DOI
[2] KNIME - the Konstanz Information Miner : Version 2.0 and Beyond, ACM SIGKDD Explorations Newsletter, Volume 11 (2009) no. 1, pp. 26-31 | DOI
[3] Missing Values and Learning of Fuzzy Rules, International Journal of Uncertainty, Fuzziness and Knowledge-Based Systems, Volume 06 (1998) no. 2, pp. 171-178 | DOI | Zbl
[4] Greedy function approximation : A gradient boosting machine., The Annals of Statistics, Volume 29 (2001) no. 5, pp. 1189-1232 | DOI | Zbl
[5] neOCampus : A Demonstrator of Connected, Innovative, Intelligent and Sustainable Campus, Intelligent Interactive Multimedia Systems and Services 2017, Volume 76, Springer (2018), pp. 482-491 | DOI
[6] Dynamic learning of the environment for eco-citizen behavior, PhD Thesis, Université Toulouse III – Paul Sabatier ; Università degli studi di Catania (2020)
[7] Estimating Missing Environmental Information by Contextual Data Cooperation, PRIMA 2019 : Principles and Practice of Multi-Agent Systems, Springer (2019), pp. 523-531 | DOI
[8] A Cooperative Multi-Agent System for Crowd Sensing Based Estimation in Smart Cities, IEEE Access, Volume 8 (2020), pp. 183051-183070 | DOI
[9] Cooperative Multi-Agent Traffic Monitoring Can Reduce Camera Surveillance, IEEE Access, Volume 11 (2023), pp. 142125-142145 | DOI
[10] Evaluating Correlations in IoT Sensors for Smart Buildings, 13th Int. Conference on Agents and Artificial Intelligence (ICAART), SCITEPRESS (2021), pp. 224-231 | DOI
[11] Time Series Analysis, Princeton University Press, 1994, 816 pages | DOI
[12] Deriving high-resolution urban air pollution maps using mobile sensor nodes, Pervasive and Mobile Computing, Volume 16 (2015), pp. 268-285 | DOI
[13] The role of big data in smart city, International Journal of Information Management, Volume 36 (2016) no. 5, pp. 748-758 | DOI
[14] Random decision forests, Proceedings of 3rd Int. Conf. on Document Analysis and Recognition, Volume 1 (1995), pp. 278-282 | DOI
[15] Structural breaks, ARIMA model and Finnish inflation forecasts, International Journal of Forecasting, Volume 17 (2001) no. 2, pp. 203 - 230 | DOI
[16] Short Term Traffic Flow Prediction for a Non Urban Highway Using Artificial Neural Network, Procedia – Social and Behavioral Sciences, Volume 104 (2013), pp. 755-764 | DOI
[17] Soft Sensor with Deep Learning for Functional Region Detection in Urban Environments, Sensors, Volume 20 (2020) no. 12, p. 3348 | DOI
[18] Computational Intelligence for Missing Data Imputation, Estimation, and Management - Knowledge Optimization Techniques, IGI Global, 2009 | DOI
[19] Machine learning methods to forecast temperature in buildings, Expert Systems with Applications, Volume 40 (2013) no. 4, pp. 1061 - 1068 | DOI
[20] Machine Learning Algorithms for Short-Term Load Forecast in Residential Buildings Using Smart Meters, Sensors and Big Data Solutions, IEEE Access, Volume 7 (2019), pp. 177874-177889 | DOI
[21] ANN-Based Soft Sensor to Predict Effluent Violations in Wastewater Treatment Plants, Sensors, Volume 19 (2019) no. 6, p. 1280 | DOI
[22] A Simple Flood Forecasting Scheme Using Wireless Sensor Networks, International Journal of Ad hoc, Sensor & Ubiquitous Computing, Volume 3 (2012) no. 1 | DOI
[23] Fusing Incomplete Multisensor Heterogeneous Data to Estimate Urban Traffic, IEEE MultiMedia, Volume 23 (2016) no. 3, pp. 56-63 | DOI
[24] A Refinement of Lasso Regression Applied to Temperature Forecasting, Procedia Computer Science, Volume 130 (2018), pp. 728-735 | DOI
[25] Evaluating the Health State of Urban Areas Using Multi-source Heterogeneous Data, 2018 IEEE 19th Int. Symposium on “A World of Wireless, Mobile and Multimedia Networks” (WoWMoM) (2018), pp. 14-22 | DOI
[26] The Radial Basis Functions – Partial Least Squares approach as a flexible non-linear regression technique, Analytica Chimica Acta, Volume 331 (1996) no. 3, pp. 177-185 | DOI
[27] Modeling for optimal probability prediction, Proceedings of the 9th Int. Conference on Machine Learning, Morgan Kaufmann, 2002, pp. 650-657
[28] Data Mining : Practical Machine Learning Tools and Techniques, Elsevier, 2011 | DOI
[29] Isotonic regression : Another look at the changepoint problem, Biometrika, Volume 88 (2001) no. 3, pp. 793-804 | DOI | Zbl
[30] Real-time forest fire detection with wireless sensor networks, Proceedings of Int. Conf. on Wireless Communications, Networking and Mobile Computing, 2005, Volume 2 (2005), pp. 1214-1217 | DOI
[31] A gradient boosting method to improve travel time prediction, Transportation Research Part C : Emerging Technologies, Volume 58 (2015), pp. 308 - 324 | DOI
[32] Granger-Causality-based air quality estimation with spatio-temporal (S-T) heterogeneous big data, Computer Communications Workshops (INFOCOM WKSHPS), IEEE (2015), pp. 612-617 | DOI
Cité par Sources :