Le développement de véhicules autonomes, capables de communiquer de pair à pair, ainsi que l’intérêt pour les solutions à la demande (par exemple, Uber, Lyft, Heetch), sont les principales motivations de cette étude. Le problème d’allocation des véhicules aux clients est d’une importance majeure dans la gestion des systèmes de transport à la demande (ODT). Il est étudié depuis des décennies, et diverses solutions ont été proposées. Les différentes familles de solutions peuvent être classées en deux catégories, centralisées et décentralisées avec dans la pratique, pour chacune ses avantages et ses inconvénients. Dans ce travail, nous visons à fournir un modèle générique pour le problème du transport à la demande en ligne avec des véhicules autonomes. Indépendant des solutions, ce modèle permet une description synthétique du problème et propose des indicateurs de qualité. Nous proposons également un modèle multi-agents dédié à l’allocation des ressources et à la planification de la flotte. Ce dernier considère des véhicules autonomes comme des agents qui communiquent dans un réseau inter-véhiculaire pour satisfaire les demandes de transport dans un système de transport à la demande selon la stratégie de résolution que l’utilisateur souhaite évaluer. Nous montrons la généricité de ce modèle en appliquant plusieurs approches d’allocation (optimisation linéaire en nombres entiers, approche gloutonne, enchères et optimisation sous contraintes distribuée) et comparons en détail leurs performances en termes de qualité de solution et d’indicateurs techniques sur des scénarios générés à partir de données réelles.
The development of autonomous vehicles, capable of peer-to-peer communication, as well as the interest in on-demand solutions (e.g., Uber, Lyft, Heetch), are the primary motivations for this study. Allocation problems are of major importance in the management of on-demand transportation systems (ODT). They have been studied for decades, and various solutions have been proposed. The solution approaches can be classified into two categories: centralized and decentralized. In practice, each has its advantages and disadvantages. In this work, we aim to provide a generic model for the problem of online on-demand transportation with autonomous vehicles and a multi-agent model dedicated to resource allocation and fleet planning. The latter considers autonomous vehicles that communicate in an inter-vehicular network to satisfy ride requests in an ODT system. We evaluate the generality of this model by applying several allocation approaches (mathematical programming, greedy heuristics, distributed constraint optimization and auctions) and compare their performances in terms of solution quality and technical and communicational indicators on synthetic scenarios generated from real data.
Accepté le :
Publié le :
Keywords: Multiagent Systems, Coordination, On demand Transport, Resource Allocation, Connected Autonomous Vehicles, Simulation.
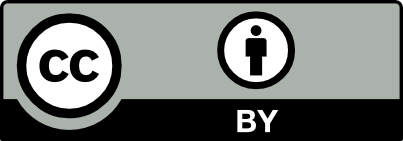
@article{ROIA_2023__4_2_169_0, author = {Alaa Daoud and Flavien Balbo and Paolo Gianessi and Gauthier Picard}, title = {AV-OLRA~: {Une} mod\'elisation g\'en\'erique pour le probl\`eme de l{\textquoteright}allocation des ressources dans le domaine du transport \`a la demande}, journal = {Revue Ouverte d'Intelligence Artificielle}, pages = {169--192}, publisher = {Association pour la diffusion de la recherche francophone en intelligence artificielle}, volume = {4}, number = {2}, year = {2023}, doi = {10.5802/roia.61}, language = {fr}, url = {https://roia.centre-mersenne.org/articles/10.5802/roia.61/} }
TY - JOUR AU - Alaa Daoud AU - Flavien Balbo AU - Paolo Gianessi AU - Gauthier Picard TI - AV-OLRA : Une modélisation générique pour le problème de l’allocation des ressources dans le domaine du transport à la demande JO - Revue Ouverte d'Intelligence Artificielle PY - 2023 SP - 169 EP - 192 VL - 4 IS - 2 PB - Association pour la diffusion de la recherche francophone en intelligence artificielle UR - https://roia.centre-mersenne.org/articles/10.5802/roia.61/ DO - 10.5802/roia.61 LA - fr ID - ROIA_2023__4_2_169_0 ER -
%0 Journal Article %A Alaa Daoud %A Flavien Balbo %A Paolo Gianessi %A Gauthier Picard %T AV-OLRA : Une modélisation générique pour le problème de l’allocation des ressources dans le domaine du transport à la demande %J Revue Ouverte d'Intelligence Artificielle %D 2023 %P 169-192 %V 4 %N 2 %I Association pour la diffusion de la recherche francophone en intelligence artificielle %U https://roia.centre-mersenne.org/articles/10.5802/roia.61/ %R 10.5802/roia.61 %G fr %F ROIA_2023__4_2_169_0
Alaa Daoud; Flavien Balbo; Paolo Gianessi; Gauthier Picard. AV-OLRA : Une modélisation générique pour le problème de l’allocation des ressources dans le domaine du transport à la demande. Revue Ouverte d'Intelligence Artificielle, Volume 4 (2023) no. 2, pp. 169-192. doi : 10.5802/roia.61. https://roia.centre-mersenne.org/articles/10.5802/roia.61/
[1] Issues in Multiagent Resource Allocation, Informatica, Volume 30 (2006), pp. 3-32 | Zbl
[2] ORNInA : A decentralized, auction-based multi-agent coordination in ODT systems, AI Communications, Volume 34 (2021) no. 1, pp. 37-53 | DOI | MR
[3] Market mechanism design for profitable on-demand transport services, Transportation Research Part B : Methodological, Volume 89 (2016), pp. 178-195 | DOI
[4] On demand transport system’s approach as a multi-agent planning problem, 2014 International Conference on Advanced Logistics and Transport (ICALT), IEEE, Tunis, Tunisia, IEEE (2014), pp. 53-58 | DOI
[5] Distributed Constraint Optimization Problems and Applications : A Survey, Journal of Artificial Intelligence Research, Volume 61 (2018), pp. 623-698 | DOI | MR | Zbl
[6] Multi-Agent Real Time Scheduling System for Taxi Companies, AAMAS (2009), pp. 29-36
[7] A Study Of Multi-Agent Based Model For Urban Intelligent Transport Systems, International Journal of Advancements in Computing Technology, Volume 4 (2012) no. 6, pp. 126-134 | DOI
[8] A dynamic cooperation modelling for improving taxi fleet efficiency, proceeding of AGILE’2012 (Jérôme Gensel; Didier Josselin; Danny Vandenbroucke, eds.), Avignon, AGILE (2012), pp. 163-168
[9] FRODO 2.0 : An Open-Source Framework for Distributed Constraint Optimization, Proceedings of the IJCAI’09 Distributed Constraint Reasoning Workshop (DCR’09), Pasadena, California, USA (2009), pp. 160-164 (https://frodo-ai.tech)
[10] Taxi Dispatch System Based on Current Demands and Real-Time Traffic Conditions, Transportation Research Record : Journal of the Transportation Research Board, Volume 1882 (2004), pp. 193-200 | DOI
[11] Evolutionary synthesis of multi-agent systems for dynamic dial-a-ride problems, international conference on Genetic and evolutionary computation conference companion - GECCO Companion ’12, ACM Press, Philadelphia, Pennsylvania, USA (2012), pp. 331-336 | DOI
[12] The influence of multi-agent cooperation on the efficiency of taxi dispatching, International conference on parallel processing and applied mathematics, Springer (2013), pp. 751-760 | DOI
[13] Quality Guarantees on K-Optimal Solutions for Distributed Constraint Optimization Problems, Proceedings of the 20th International Joint Conference on Artifical Intelligence (IJCAI’07), Morgan Kaufmann Publishers Inc., San Francisco, CA, USA (2007), p. 1446–1451
[14] Simulating demand-responsive transportation : a review of agent-based approaches, Transport Reviews, Volume 35 (2015) no. 4, pp. 404-421 | DOI
[15] A multi-agent approach to Intelligent Transportation Systems modeling with combinatorial auctions, Expert Systems with Applications, Volume 41 (2014) no. 15, pp. 6622-6633 | DOI
[16] A Collaborative Multiagent Taxi-Dispatch System, IEEE Transactions on Automation Science and Engineering, Volume 7 (2010) no. 3, pp. 607-616 | DOI
[17] Managing Autonomous Mobility on Demand Systems for Better Passenger Experience, PRIMA 2015 : Principles and Practice of Multi-Agent Systems (Lecture Notes in Computer Science), Volume 9387, Springer, Cham (2015), pp. 20-35 | DOI
[18] Multiagent systems : Algorithmic, game-theoretic, and logical foundations, Cambridge University Press, 2009
[19] Flexible Multi-Agent System for Distributed Coordination, Transportation & Localisation, Proceedings of the 17th International Conference on Autonomous Agents and MultiAgent Systems (AAMAS ’18), International Foundation for Autonomous Agents and Multiagent Systems, Richland, SC (2018), p. 1832–1834
[20] Central Decision Intellective Taxi System and Multi Ride Algorithm, International Conference on Artificial Intelligence, Automation and Control Technologies (AIACT ’17), ACM, New York, NY, USA (2017), 5, pp. 1-6 (event-place : Wuhan, China) | DOI
[21] Generic model for resource allocation in transportation. Application to urban parking management, Transportation Research Part C : Emerging Technologies, Volume 71 (2016), pp. 538-554 | DOI
[22] Distributed stochastic search and distributed breakout : properties, comparison and applications to constraint optimization problems in sensor networks, Artificial Intelligence, Volume 161 (2005) no. 1, pp. 55-87 | DOI | MR | Zbl
Cité par Sources :