La négociation automatique suscite un intérêt croissant dans la recherche en intelligence artificielle. Bien que de nombreuses hypothèses aient été explorées, les résultats proposés ne sont pas satisfaisants vis-à-vis de certaines applications. C’est notamment le cas de l’affacturage, qui propose un intéressant défi de par ses spécificités, notamment l’impossibilité de borner la négociation en termes de temps, mais aussi la taille des domaines de négociation, potentiellement infinis. Les méthodes de Monte-Carlo constituent un outil intéressant pour aborder cette application en raison de leur efficacité face à ce type d’hypothèses.
Dans cet article, nous décrivons un agent de négociation automatique, le Monte-Carlo Negotiating Agent (MoCaNA) dont la stratégie d’offre s’appuie sur la recherche arborescente de Monte-Carlo. MoCaNA est doté de méthodes de modélisation du comportement de l’opposant. Il est capable de négocier sur des domaines de négociation incluant des attributs discrets et continus, linéaires ou non, dans un contexte où aucune date butoir n’est spécifiée. Nous confrontons MoCaNA aux agents de l’ANAC 2014 et à d’autres agents capables de négocier sans date butoir, sur des domaines de négociation différents. Il se montre capable de surpasser ou égaler tous les agents dans un domaine sans date butoir et la majorité des finalistes de l’ANAC dans un domaine avec date butoir.
Automated negotiation is of growing interest in artificial intelligence research. While research has focused numerous contexts, some applications have not benefited from these advances. This is particularly the case for factoring, which offers an interesting challenge due to its specificities, notably the impossibility to limit the negotiation in terms of time, and negotiation on vast, even infinite domains. Monte-Carlo methods are an interesting tool for solving this problem because of their effectiveness with such hypotheses.
In this paper, we introduce a Monte Carlo Negotiating Agent (MoCaNA) whose bidding strategy relies on Monte Carlo Tree Search. We endow MoCaNA with opponent modeling techniques for bidding strategy and utility. MoCaNA can negotiate on continuous domains and in a context where no bound is specified. We confront MoCaNA with both the finalists of ANAC 2014 and to agents that are able to negotiate without bounds on different negotiation domains. MoCaNA outperforms or ties all the agents in a domain without bound and the majority of the ANAC finalists in a domain with a bound.
Révisé le :
Accepté le :
Publié le :
Keywords: Monte Carlo, Automated Negotiation, Agent
Cédric L.R. Buron 1 ; Zahia Guessoum 2, 3 ; Sylvain Ductor 4 ; Olivier Roussel 5
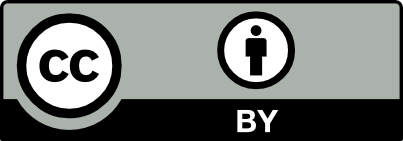
@article{ROIA_2022__3_5-6_645_0, author = {C\'edric L.R. Buron and Zahia Guessoum and Sylvain Ductor and Olivier Roussel}, title = {MoCaNA, un agent de n\'egociation automatique utilisant la recherche arborescente de {Monte-Carlo}}, journal = {Revue Ouverte d'Intelligence Artificielle}, pages = {645--669}, publisher = {Association pour la diffusion de la recherche francophone en intelligence artificielle}, volume = {3}, number = {5-6}, year = {2022}, doi = {10.5802/roia.46}, language = {fr}, url = {https://roia.centre-mersenne.org/articles/10.5802/roia.46/} }
TY - JOUR AU - Cédric L.R. Buron AU - Zahia Guessoum AU - Sylvain Ductor AU - Olivier Roussel TI - MoCaNA, un agent de négociation automatique utilisant la recherche arborescente de Monte-Carlo JO - Revue Ouverte d'Intelligence Artificielle PY - 2022 SP - 645 EP - 669 VL - 3 IS - 5-6 PB - Association pour la diffusion de la recherche francophone en intelligence artificielle UR - https://roia.centre-mersenne.org/articles/10.5802/roia.46/ DO - 10.5802/roia.46 LA - fr ID - ROIA_2022__3_5-6_645_0 ER -
%0 Journal Article %A Cédric L.R. Buron %A Zahia Guessoum %A Sylvain Ductor %A Olivier Roussel %T MoCaNA, un agent de négociation automatique utilisant la recherche arborescente de Monte-Carlo %J Revue Ouverte d'Intelligence Artificielle %D 2022 %P 645-669 %V 3 %N 5-6 %I Association pour la diffusion de la recherche francophone en intelligence artificielle %U https://roia.centre-mersenne.org/articles/10.5802/roia.46/ %R 10.5802/roia.46 %G fr %F ROIA_2022__3_5-6_645_0
Cédric L.R. Buron; Zahia Guessoum; Sylvain Ductor; Olivier Roussel. MoCaNA, un agent de négociation automatique utilisant la recherche arborescente de Monte-Carlo. Revue Ouverte d'Intelligence Artificielle, Post-actes des Journées Francophones sur les Systèmes Multi-Agents (JFSMA 2018-2019-2020), Volume 3 (2022) no. 5-6, pp. 645-669. doi : 10.5802/roia.46. https://roia.centre-mersenne.org/articles/10.5802/roia.46/
[1] Exploring the Strategy Space of Negotiating Agents : A Framework for Bidding, Learning and Accepting in Automated Negotiation, Ph. D. Thesis, Delft University of Technology (2016) | DOI
[2] The automated negotiating agents competition, 2010–2015, AI Magazine, Volume 36 (2015) no. 4, pp. 115-118 | DOI
[3] Learning about the opponent in automated bilateral negotiation : a comprehensive survey of opponent modeling techniques, Autonomous Agents and Multi-Agent Systems, Volume 20 (2015) no. 1, pp. 1-50 | DOI
[4] , AAMAS ’13 (2013), pp. 715-722 http://dl.acm.org/citation.cfm?id=2484920.2485033
[5] A Tit for Tat Negotiation Strategy for Real-Time Bilateral Negotiation, Complex Automated Negotiations : Theories, Models, and Software Competitions, Volume 435, Springer Berlin Heidelberg, 2013, pp. 229-233 | DOI
[6] A Survey of Monte Carlo Tree Search Methods, IEEE Transactions on Computational Intelligence and AI in games, Volume 4 (2012) no. 1, pp. 1-43 | DOI
[7] , Multiagent System Technologies : 10th German Conference Proceedings (2012), pp. 68-82 | DOI
[8] , International conference on computers and games (2006), pp. 72-83 | DOI
[9] Crowdfunding Platforms in Invoice Trading as Alternative Financial Markets, Roczniki Kolegium Analiz Ekonomicznych/Szkoła Główna Handlowa, Volume 49 (2018), pp. 455-464
[10] , 2008 International Symposium on Electronic Commerce and Security (2008) | DOI
[11] Negotiation decision functions for autonomous agents, Robotics and Autonomous Systems, Volume 24 (1998) no. 3-4, pp. 159-182 | DOI
[12] The Fifth Automated Negotiating Agents Competition (ANAC 2014), Recent Advances in Agent-based Complex Automated Negotiation (Naoki Fukuta; Takayuki Ito; Minjie Zhang; Katsuhide Fujita; Valentin Robu, eds.), Springer International Publishing, Cham, 2016, pp. 211-224 | DOI
[13] Recent Advances in Agent-based Complex Automated Negotiation (Naoki Fukuta; Takayuki Ito; Minjie Zhang; Katsuhide Fujita; Valentin Robu, eds.), Studies in Computational Intelligence, 638, Springer International Publishing, 2016 | DOI
[14] Agent Smith : Opponent Model Estimation in Bilateral Multi-issue Negotiation, New Trends in Agent-Based Complex Automated Negotiations (Takayuki Ito; Minjie Zhang; Valentin Robu; Shaheen Fatima; Tokuro Matsuo, eds.), Springer Berlin Heidelberg, Berlin, Heidelberg, 2012, pp. 167-174 | DOI
[15] Monte-Carlo tree search and rapid action value estimation in computer Go, Artificial Intelligence, Volume 175 (2011) no. 11, pp. 1856-1875 | DOI | MR
[16] , IC-AI (2009), pp. 605-610
[17] Jama : A Java matrix package, 2000 (http://math. nist. gov/javanumerics/jama)
[18] , Proceedings of the 7th International Joint Conference on Autonomous Agents and Multiagent Systems, Volume 1 (2008), pp. 331-338 http://dl.acm.org/citation.cfm?id=1402383.1402433
[19] Non-Zero-Sum Stochastic Games, Handbook of Dynamic Game Theory (Tamer Basar; Georges Zaccour, eds.), Springer International Publishing, Cham, 2016, pp. 1-64 | DOI
[20] , AAMAS ’17 (2017), pp. 371-379 http://dl.acm.org/citation.cfm?id=3091125.3091183
[21] , Machine Learning : ECML 2006 (2006), pp. 282-293 | DOI
[22] Genius : an integrated environment for supporting the design of generic automated negotiators, Computational Intelligence, Volume 30 (2014) no. 1, pp. 48-70 | DOI | MR
[23] , Vingt-sixièmes journées francophones sur les systèmes multi-agents (2018), pp. 75-84
[24] The bargaining problem, Econometrica : Journal of the econometric society (1950), pp. 155-162 | DOI | MR | Zbl
[25] A course in game theory, MIT press, 1994
[26] Rapport annuel des délais de paiement en 2019 (2020) (Technical report)
[27] Negotiation Behavior, Academic Press, 1981
[28] Gaussian processes for machine learning, MIT Press, 2006
[29] Learning-based automated negotiation between shipper and forwarder, Computers & Industrial Engineering, Volume 51 (2006) no. 3, pp. 464 -481 http://www.sciencedirect.com/science/article/pii/S0360835206001070 Special Issue on Selected Papers from the 34th. International Conference on Computers and Industrial Engineering (ICC&IE) | DOI
[30] , Proceedings of the 20th Conference on Uncertainty in Artificial Intelligence (UAI ’04) (2004), pp. 470-478 http://dl.acm.org/citation.cfm?id=1036843.1036900
[31] Distributed rational decision making, Multiagent systems : a modern approach to distributed artificial intelligence (1999), pp. 201-258
[32] et al. Mastering the game of Go with deep neural networks and tree search, Nature, Volume 529 (2016) no. 7587, pp. 484-489 | DOI
[33] The apache commons mathematics library, online, 2016 (see https://commons.apache.org/proper/commons-math/)
[34] , IJCAI’11 (2011), pp. 432-438 | DOI
Cité par Sources :