Le déficit d’explicabilité des techniques d’apprentissage machine (AM) pose des problèmes opérationnels, juridiques et éthiques. Un des principaux objectifs de notre projet est de fournir des explications éthiques des sorties générées par une application fondée sur de l’AM, considérée comme une boîte noire. La première étape de ce projet, présentée dans cet article, consiste à montrer que la validation de ces boîtes noires diffère épistémologiquement de celle mise en place dans le cadre d’une modélisation mathématique et causale d’un phénomène physique. La différence majeure est qu’une méthode d’AM ne prétend pas représenter une causalité entre les paramètres d’entrées et ceux de sortie. Après avoir proposé une clarification et une adaptation des notions d’interprétabilité et d’explicabilité telles qu’on les rencontre dans la littérature déjà abondante sur le sujet, nous montrons dans cet article l’intérêt de mettre en œuvre les distinctions épistémologiques entre les différentes fonctions épistémiques d’un modèle, d’une part, et entre la fonction épistémique et l’usage d’un modèle, d’autre part. Enfin, la dernière partie de cet article présente nos travaux en cours sur l’évaluation d’une explication, qui peut être plus persuasive qu’informative, ce qui peut ainsi causer des problèmes d’ordre éthique.
The lack of explainability of machine learning (ML) techniques poses operational, legal and ethical problems. One of the main goals of our project is to provide ethical explanations of the outputs generated by an ML-based application, considered as a black box. The first step of this project, presented in this paper, is to show that the validation of these black boxes differs epistemologically from that implemented in the framework of a mathematical and causal modeling of a physical phenomenon. The major difference is that an ML method does not claim to represent causality between input and output parameters. After having provided a clarification and an adaptation of the notions of interpretability and explainability as found in the already abundant literature on the subject, we show in this article the fruitfulness of implementing the epistemological distinctions between the different epistemic functions of a model, on the one hand, and between the epistemic function and the use of a model, on the other hand. Finally, the last part of this article presents our current work on the evaluation of an explanation, which can be more persuasive than informative, and which can therefore raise ethical problems.
Révisé le :
Accepté le :
Publié le :
Keywords: Machine learning, interpretability, explainability, epistemology.
Christophe Denis 1 ; Franck Varenne 1
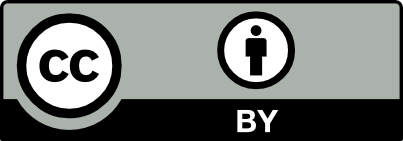
@article{ROIA_2022__3_3-4_287_0, author = {Christophe Denis and Franck Varenne}, title = {Interpr\'etabilit\'e et explicabilit\'e de ph\'enom\`enes pr\'edits par de l{\textquoteright}apprentissage machine}, journal = {Revue Ouverte d'Intelligence Artificielle}, pages = {287--310}, publisher = {Association pour la diffusion de la recherche francophone en intelligence artificielle}, volume = {3}, number = {3-4}, year = {2022}, doi = {10.5802/roia.32}, language = {fr}, url = {https://roia.centre-mersenne.org/articles/10.5802/roia.32/} }
TY - JOUR AU - Christophe Denis AU - Franck Varenne TI - Interprétabilité et explicabilité de phénomènes prédits par de l’apprentissage machine JO - Revue Ouverte d'Intelligence Artificielle PY - 2022 SP - 287 EP - 310 VL - 3 IS - 3-4 PB - Association pour la diffusion de la recherche francophone en intelligence artificielle UR - https://roia.centre-mersenne.org/articles/10.5802/roia.32/ DO - 10.5802/roia.32 LA - fr ID - ROIA_2022__3_3-4_287_0 ER -
%0 Journal Article %A Christophe Denis %A Franck Varenne %T Interprétabilité et explicabilité de phénomènes prédits par de l’apprentissage machine %J Revue Ouverte d'Intelligence Artificielle %D 2022 %P 287-310 %V 3 %N 3-4 %I Association pour la diffusion de la recherche francophone en intelligence artificielle %U https://roia.centre-mersenne.org/articles/10.5802/roia.32/ %R 10.5802/roia.32 %G fr %F ROIA_2022__3_3-4_287_0
Christophe Denis; Franck Varenne. Interprétabilité et explicabilité de phénomènes prédits par de l’apprentissage machine. Revue Ouverte d'Intelligence Artificielle, Post-actes de la Conférence Nationale en Intelligence Artificielle (CNIA 2018-2020), Volume 3 (2022) no. 3-4, pp. 287-310. doi : 10.5802/roia.32. https://roia.centre-mersenne.org/articles/10.5802/roia.32/
[1] The End of Theory : The Data Deluge Makes the Scientific Method Obsolete, Wired, Volume 16 (2008) no. 7
[2] Novum organum, 1620
[3] L’art poétique, Flammarion, 1998
[4] Reseaux de Neurones Convolutifs pour la Caractérisation d’Anomalies Magnétiques, 12ème colloque GEOFCAN, Grenoble, France (2021)
[5] Réseaux de Neurones Convolutifs pour la Caractérisation d’Anomalies Magnétiques, CNIA 2021 : Conférence Nationale en Intelligence Artificielle (2021), pp. 84-90
[6] En attendant les robots. Enquête sur le travail du clic, Le Seuil, 2019
[7] Algorithmic Information Theory, Cambridge Tracts in Theoretical Computer Science, Cambridge University Press, Cambridge, 1987 | DOI
[8] Embedded Constrained Feature Construction for High-Energy Physics Data Classification, 33rd Annual Conference on Neural Information Processing Systems (2019)
[9] Interprétabilité et explicabilité pour l’apprentissage machine : entre modèles descriptifs, modèles prédictifs et modèles causaux. Une nécessaire clarification épistémologique, National (French) Conference on Artificial Intelligence (CNIA) – Artificial Intelligence Platform (PFIA) (2019), pp. 60-68
[10] Le mythe de la singularité. Faut-il craindre l’Intelligence Articielle ?, Le Seuil, 2017
[11] Comment faire sortir l’intelligence artificielle des labos ?, Les échos, 2019
[12] Automatic Chemical Design Using a Data-Driven Continuous Representation of Molecules, ACS Central Science, Volume 4 (2018), pp. 268-276 | DOI
[13] Explaining Explanations : An Overview of Interpretability of Machine Learning, 2018 IEEE 5th International Conference on Data Science and Advanced Analytics (DSAA) (2018), pp. 80-89 | DOI
[14] The Promise and Peril of Human Evaluation for Model Interpretability, Thirsty-first Conference on Neural Information Processing Systems, NIPS 2017 (2017)
[15] Ethics guidelines for trustworthy AI (2019) (Technical report)
[16] Understanding climate change with statistical downscaling and machine learning, Synthese, Volume 199 (2021), pp. 1877-1897 | DOI | MR
[17] What Uncertainties Do We Need in Bayesian Deep Learning for Computer Vision ?, Thirsty-first Conference on Neural Information Processing Systems, NIPS 2017 (2017), pp. 5580-5590
[18] The Mythos of Model Interpretability, Communications of the ACM, Volume 61 (2018) no. 10, pp. 36-43 | DOI
[19] Interpretability in Machine Learning – Principles and Practice, Fuzzy Logic and Applications (2013), pp. 15-21 | DOI
[20] Explanation and Abductive Inference, The Oxford Handbook of Thinking and Reasoning (2012) | DOI
[21] Understanding deep convolutional networks, Philosophical Transactions of the Royal Society A : Mathematical, Physical and Engineering Sciences, Volume 374 (2016) no. 2065 | DOI
[22] Explanation in artificial intelligence : Insights from the social sciences, Artificial Intelligence, Volume 267 (2019), pp. 1-38 | DOI | MR | Zbl
[23] Matter, Mind and Models, Proc. of the International Federation of Information Processing Congress (1965)
[24] Explaining Explanations in AI, FAT* ’19 : Proceedings of the Conference on Fairness, Accountability, and Transparency (2019) | DOI
[25] Interpretable Machine Learning – A Guide for Making Black Box Models Explainable (2019) (https://christophm.github.io/interpretable-ml-book/)
[26] Reconstructing Reality : Models, Mathematics, and Simulations, Oxford University Press, 2015 | DOI
[27] The Agnostic Structure of Data Science Methods (2021) (https://arxiv.org/abs/2101.12150)
[28] Verification and Validation in Scientific Computing, Cambridge University Press, 2010 | DOI
[29] A perspective on machine learning in turbulent flows, Journal of Turbulence, Volume 21 (2020) no. 9-10, pp. 567-584 | DOI | MR
[30] Model-Free Prediction of Large Spatiotemporally Chaotic Systems from Data : A Reservoir Computing Approach, Phys. Rev. Lett., Volume 120 (2018), 024102 | DOI
[31] Causality : Models, Reasoning, and Inference, Cambridge University Press, 2000
[32] Machine Learning Has Become Alchemy, Thirsty-first Conference on Neural Information Processing Systems, NIPS 2017 (2017)
[33] Philosophie du langage (et de l’esprit), Folio-Essais, Gallimard, 2008
[34] Understanding Machine Learning : From Theory to Algorithms, Cambridge University Press, 2014 | DOI
[35] Prédire n’est pas expliquer, Flammarion, 1991
[36] Présentation, Réseaux, Volume 188 (2014) no. 6, pp. 9-21 | DOI
[37] Modèles et simulations dans l’enquête scientifique : variétés traditionnelles et mutations contemporaines, Modéliser & Simuler. Épistémologies et pratiques de la modélisation et de la simulation, Tome I (F. Varenne; M. Silberstein, eds.), Matériologiques, 2013
[38] From Models to Simulations, Routledge, 2018 | DOI
[39] Donner un sens à l’intelligence artificielle : Pour une stratégie nationale et européenne (2018)
[40] Modeling polypharmacy side effects with graph convolutional networks, Bioinformatics, Volume 34 (2018) no. 13, p. i457-i466 | DOI
Cité par Sources :