Cet article a pour objet la détection de plantes à partir d’images aériennes capturées par drones. Une méthode efficace de détection bénéficierait autant aux agriculteurs qui souhaitent connaître l’état de leurs cultures et en prédire le rendement qu’aux agronomes pour faciliter l’acquisition de données expérimentales et en réduire le coût.
Nous proposons une méthode en deux étapes avec, d’abord, un apprentissage non supervisé, puis la mise en œuvre d’un système multi-agent faiblement paramétré. Dans un premier temps, un clustering estime la position des rangs et des plantes en s’appuyant sur des contraintes géométriques connues a priori, à savoir que les rangs de cultures sont globalement équidistants entre eux et qu’il en est de même pour les plantes au sein d’un rang. Dans un second temps, le système multi-agent raffine l’estimation fournie par l’étape de clustering et isole chaque plante.
Nous démontrons les performances de la méthode sur des tâches de comptage de plants de tournesols. Les résultats obtenus sont comparables à ceux de l’état de l’art sur les problèmes faciles et nettement supérieurs sur les problèmes difficiles. Nous présentons ensuite des résultats sur l’identification de gradients de densité dans un champ en fonction de la répartition des plantes qui ont levées.
Une contribution importante de ce travail concerne également la mise au point d’un outil de génération de champs virtuels avec le moteur de jeu Unity. Il devient ainsi possible de générer facilement des jeux de données réalistes correspondant à des situations diverses ce qui permet de contourner la difficulté d’obtenir des jeux de données étiquetés.
This paper focuses on the detection of plants from aerial images captured by drones. An efficient detection method would benefit both farmers who wish to know the state of their crops and predict their yield and agronomists to facilitate the acquisition of experimental data and reduce the cost.
We propose a two-step method with, first, an unsupervised learning, then the implementation of a weakly parameterized multi-agent system. In the first step, a clustering system estimates the position of rows and plants based on a priori known geometric constraints, namely that crop rows are globally equidistant from each other and that the same is true for plants within a row. In a second step, the multi-agent system refines the estimation provided by the clustering step and isolates each plant.
We demonstrate the performance of the method on sunflower plant counting tasks. The results obtained are comparable to those of the state of the art on easy problems and significantly better on hard problems. We then present results on the identification of density gradients in a field based on the distribution of emerged plants.
An important contribution of this work also concerns the development of a tool for generating virtual fields with the game engine Unity. It is thus possible to easily generate realistic datasets corresponding to various situations, thus overcoming the difficulty of obtaining labelled datasets.
Révisé le :
Accepté le :
Publié le :
Keywords: Unsupervised learning, multi-agent system, analysis of images from UAVs.
Eliott Jacopin 1 ; Antoine Cornuéjols 1 ; Christine Martin 1 ; Farzaneh Kazemipour 2 ; Christophe Sausse 2
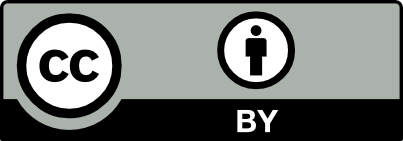
@article{ROIA_2021__2_1_123_0, author = {Eliott Jacopin and Antoine Cornu\'ejols and Christine Martin and Farzaneh Kazemipour and Christophe Sausse}, title = {D\'etection automatique de plantes au sein d{\textquoteright}images a\'eriennes de~champs par apprentissage non supervis\'e et approche multi-agents}, journal = {Revue Ouverte d'Intelligence Artificielle}, pages = {123--156}, publisher = {Association pour la diffusion de la recherche francophone en intelligence artificielle}, volume = {2}, number = {1}, year = {2021}, doi = {10.5802/roia.12}, language = {fr}, url = {https://roia.centre-mersenne.org/articles/10.5802/roia.12/} }
TY - JOUR AU - Eliott Jacopin AU - Antoine Cornuéjols AU - Christine Martin AU - Farzaneh Kazemipour AU - Christophe Sausse TI - Détection automatique de plantes au sein d’images aériennes de champs par apprentissage non supervisé et approche multi-agents JO - Revue Ouverte d'Intelligence Artificielle PY - 2021 SP - 123 EP - 156 VL - 2 IS - 1 PB - Association pour la diffusion de la recherche francophone en intelligence artificielle UR - https://roia.centre-mersenne.org/articles/10.5802/roia.12/ DO - 10.5802/roia.12 LA - fr ID - ROIA_2021__2_1_123_0 ER -
%0 Journal Article %A Eliott Jacopin %A Antoine Cornuéjols %A Christine Martin %A Farzaneh Kazemipour %A Christophe Sausse %T Détection automatique de plantes au sein d’images aériennes de champs par apprentissage non supervisé et approche multi-agents %J Revue Ouverte d'Intelligence Artificielle %D 2021 %P 123-156 %V 2 %N 1 %I Association pour la diffusion de la recherche francophone en intelligence artificielle %U https://roia.centre-mersenne.org/articles/10.5802/roia.12/ %R 10.5802/roia.12 %G fr %F ROIA_2021__2_1_123_0
Eliott Jacopin; Antoine Cornuéjols; Christine Martin; Farzaneh Kazemipour; Christophe Sausse. Détection automatique de plantes au sein d’images aériennes de champs par apprentissage non supervisé et approche multi-agents. Revue Ouverte d'Intelligence Artificielle, Volume 2 (2021) no. 1, pp. 123-156. doi : 10.5802/roia.12. https://roia.centre-mersenne.org/articles/10.5802/roia.12/
[1] Blender - a 3D modelling and rendering package (2018) http://www.blender.org | DOI
[2] L-Py : An L-System Simulation Framework for Modeling Plant Architecture Development Based on a Dynamic Language, Frontiers in Plant Science, Volume 3 (2012), 76, 20 pages https://www.frontiersin.org/articles/10.3389/fpls.2012.00076/full | DOI
[3] Satellite-based assessment of yield variation and its determinants in smallholder African systems, Proceedings of the National Academy of Sciences, Volume 114 (2017) no. 9, pp. 2189-2194 | DOI
[4] Digital Count of Corn Plants Using Images Taken by Unmanned Aerial Vehicles and Cross Correlation of Templates, Agronomy, Volume 10 (2020) no. 4, 469, 16 pages | DOI
[5] Digital counts of maize plants by unmanned aerial vehicles (UAVs), Remote sensing, Volume 9 (2017) no. 6, 544, 15 pages | DOI
[6] Support vector machines for crop/weeds identification in maize fields, Expert Systems with Applications, Volume 39 (2012) no. 12, pp. 11149-11155 | DOI
[7] Automatic segmentation of relevant textures in agricultural images, Computers and Electronics in Agriculture, Volume 75 (2011) no. 1, 76, pp. 75-83 | DOI
[8] A guidance directrix approach to vision-based vehicle guidance systems, Computers and Electronics in Agriculture, Volume 43 (2004) no. 3, pp. 179-195 https://linkinghub.elsevier.com/retrieve/pii/S0168169904000286 | DOI
[9] An Annotation Saved is an Annotation Earned : Using Fully Synthetic Training for Object Instance Detection (2019) (http://arxiv.org/abs/1902.09967) | DOI
[10] Multi-agent Systems in Remote Sensing Image Analysis, Proceedings of the 11th International Conference on Agents and Artificial Intelligence - Volume 1 : ICAART 2019 (2019), pp. 178-185 | DOI
[11] Using Agents and Unsupervised Learning for Counting Objects in Images with Spatial Organization :, Proceedings of the 13th International Conference on Agents and Artificial Intelligence (2021), 544, pp. 688-697 https://www.scitepress.org/DigitalLibrary/Link.aspx?doi=10.5220/0010228706880697 | DOI
[12] Training a performant object detection ML model on synthetic data using Unity Perception tools, https://blogs.unity3d.com/2020/09/17/training-a-performant-object-detection-ml-model-on-synthetic-data-using-unity-computer-vision-tools/, 2020, 931
[13] Mapping smallholder yield heterogeneity at multiple scales in Eastern Africa, Remote Sensing, Volume 9 (2017) no. 9, 931, 15 pages
[14] Unity : A General Platform for Intelligent Agents (2020) (http://arxiv.org/abs/1809.02627)
[15] Algorithmic Botany via Lindenmayer Systems in Blender, Bachelor Thesis, TU Wien, Faculty of Computer Science (2017)
[16] Algorithmic Beauty of Plants, Springer New York, New York, 1990 http://public.ebookcentral.proquest.com/choice/publicfullrecord.aspx?p=3078014 (OCLC : 958528770) | DOI
[17] Eyes in the Sky, Boots on the Ground : Assessing Satellite-and Ground-Based Approaches to Crop Yield Measurement and Analysis, American Journal of Agricultural Economics, Volume 102 (2020) no. 1, pp. 202-219 | DOI
[18] Synthetic Data for Deep Learning (2019) (http://arxiv.org/abs/1909.11512)
[19] Designing Transparent and Autonomous Intelligent Vision Systems, Proceedings of the 11th International Conference on Agents and Artificial Intelligence - Volume 2 : ICAART 2019 (2019), pp. 850-856 | DOI
[20] A Threshold Selection Method from Gray-Level Histograms, IEEE Transactions on Systems, Man, and Cybernetics, Volume 9 (1979) no. 1, pp. 62-66 (Conference Name : IEEE Transactions on Systems, Man, and Cybernetics) | DOI
[21] Selecting patterns and features for between-and within-crop-row weed mapping using UAV-imagery, Expert Systems with Applications, Volume 47 (2016), pp. 85-94 | DOI
[22] An image synthesizer, ACM SIGGRAPH Computer Graphics, Volume 19 (1985) no. 3, pp. 287-296 | DOI
[23] Tools for Procedural Generation of Plants in Virtual Scenes, Computational Science - ICCS 2009 (Lecture Notes in Computer Science) (2009), pp. 801-810 | DOI
[24] Counting plants using deep learning, 2017 IEEE global conference on signal and information processing (GlobalSIP) (2017), 469, pp. 1344-1348 | DOI
[25] CAD2RL : Real Single-Image Flight without a Single Real Image (2017) (http://arxiv.org/abs/1611.04201)
[26] Assessing yield and fertilizer response in heterogeneous smallholder fields with UAVs and satellites, Field Crops Research, Volume 221 (2018), pp. 98-107 | DOI
[27] Domain Randomization for Transferring Deep Neural Networks from Simulation to the Real World (2017) (http://arxiv.org/abs/1703.06907)
[28] Unity 2019.4.15, 2020 (https://unity.com/)
[29] Unity Perception Package, https://github.com/Unity-Technologies/com.unity.perception, 2020
[30] Object Detection in 20 Years : A Survey (2019) (http://arxiv.org/abs/1905.05055) | DOI
Cité par Sources :